In recent years, the insurance industry has witnessed a significant transformation driven by technological advancements, particularly in data analytics and decentralization.
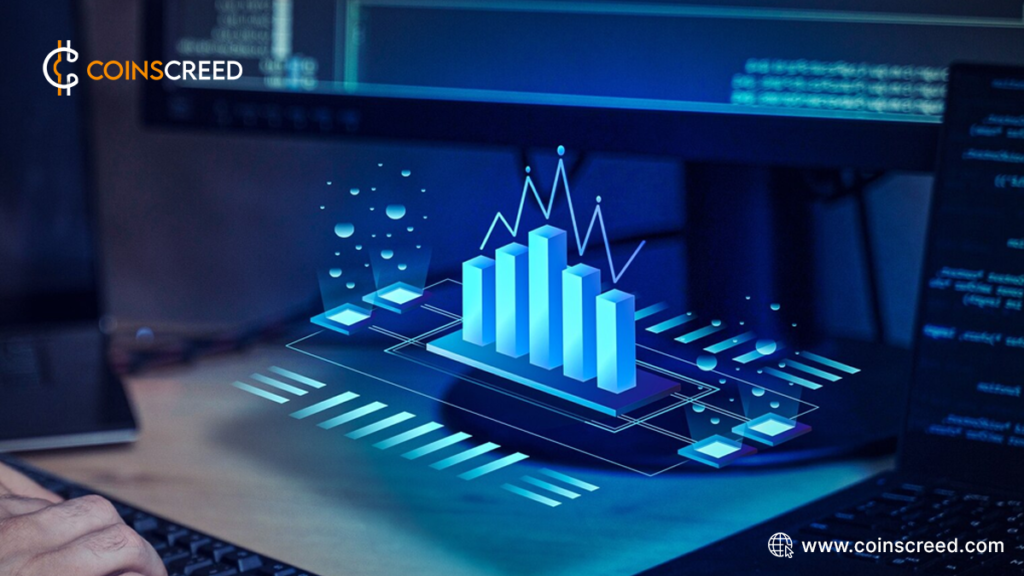
One area where these innovations are making a profound impact is underwriting, the process through which insurers assess risks and determine premiums for policies.
Decentralized insurance underwriting, empowered by data analytics, offers a paradigm shift in how risks are evaluated, policies are priced, and claims are processed. This article explores the evolution of decentralized insurance underwriting and the pivotal role of data analytics in shaping its future.
Understanding Decentralized Insurance Underwriting
Decentralized insurance underwriting refers to a system where distributed networks or platforms replace or augment the conventional centralized decision-making process. In this model, data is collected from various sources, analyzed using advanced algorithms, and utilized to assess risks and determine policy terms autonomously or semi-autonomously. Blockchain technology often serves as the backbone of decentralized insurance platforms, enabling data transparency, security, and immutability.
Data Analytics in Insurance
Historically, insurance underwriting relied heavily on actuarial tables, historical data, and manual assessments by underwriters. However, the proliferation of digital technologies and the exponential growth of data have transformed this landscape.Â
Today, insurers have access to vast amounts of structured and unstructured data, including customer demographics, behavioral data, IoT-generated data, social media feeds, and more.
Data analytics tools, such as machine learning algorithms, predictive modeling, and natural language processing, have empowered insurers to derive actionable insights from this wealth of data.Â
These insights enable more accurate risk assessment, personalized pricing, fraud detection, and enhanced customer experiences.
Advantages of Leveraging Data and Analytics in Decentralized Insurance Underwriting
Leveraging data and analytics in decentralized insurance underwriting offers many advantages, revolutionizing traditional underwriting processes and enhancing overall efficiency and effectiveness. Here are some key benefits:
Improved Risk Assessment Accuracy
Data analytics enable insurers to gather and analyze vast amounts of structured and unstructured data from diverse sources. Insurers can develop more accurate risk models by incorporating demographic information, historical claims data, IoT device data, and social media activity. This allows for better identification and assessment of risks associated with individual policyholders or properties, leading to more precise underwriting decisions.
Enhanced Predictive Capabilities
Advanced analytics techniques like machine learning and predictive modeling empower insurers to more accurately anticipate future trends and events. By analyzing historical data patterns and identifying correlations, insurers can predict potential risks and claims trends, enabling proactive risk mitigation strategies and better-informed underwriting decisions.
Personalized Underwriting Solutions
Data analytics enable insurers to gain deeper insights into customer behavior, preferences, and risk profiles. By leveraging customer data, insurers can offer personalized underwriting solutions tailored to individual policyholders’ needs and circumstances. This personalized approach enhances customer satisfaction, improves retention rates, and strengthens customer loyalty.
Efficiency and Automation
Data-driven underwriting processes streamline operations and reduce manual intervention, increasing efficiency and cost savings. Automating repetitive tasks like data entry and risk assessment frees up underwriters’ time to focus on more complex cases and strategic decision-making. Additionally, using predictive analytics and machine learning algorithms enables faster processing of applications and claims, improving overall operational efficiency.
Fraud Detection and Prevention
Data analytics play a crucial role in fraud detection and prevention in insurance underwriting. By analyzing patterns and anomalies in claim data, insurers can identify potentially fraudulent activities and take proactive measures to mitigate risks. Advanced analytics techniques, such as anomaly detection and network analysis, help insurers detect fraudulent behavior early, reducing losses and protecting the integrity of the insurance pool.
Real-time Insights and Decision-making
Data analytics give insurers real-time insights into market trends, customer behavior, and emerging risks. By leveraging these insights, insurers can make informed underwriting decisions quickly and adapt to changing market conditions more effectively. Real-time analytics capabilities enable insurers to stay ahead of the competition and seize opportunities for growth and innovation.
Compliance and Regulatory Reporting
Data analytics facilitate compliance with regulatory requirements and reporting standards by providing insurers with robust data management and reporting capabilities. Insurers can reduce the risk of regulatory penalties and fines by automating compliance processes and ensuring data accuracy and integrity. Advanced analytics tools also enable insurers to conduct comprehensive audits and demonstrate compliance with regulatory mandates more efficiently.
Data-driven Business Strategies
Leveraging data and analytics in decentralized insurance underwriting enables insurers to develop data-driven business strategies and identify new market opportunities. By analyzing customer data, market trends, and competitive intelligence, insurers can identify areas for growth, optimize pricing strategies, and develop innovative products and services that meet evolving customer needs.
Challenges Associated with Leveraging Data and Analytics in Decentralized Insurance Underwriting
While leveraging data and analytics in decentralized insurance underwriting offers numerous advantages, it also presents several challenges that insurers must navigate to realize the full potential of these technologies. Some of the key challenges include:
Data Quality and Integration
One of the primary challenges insurers face is ensuring the quality and consistency of the data used for underwriting purposes. Data from various sources may need to be completed, accurate, or consistent, making it challenging to derive meaningful insights. Additionally, integrating data from disparate sources into a cohesive underwriting process can be complex and time-consuming, requiring robust data management and integration strategies.
Data Privacy and Security
Insurers must adhere to stringent data privacy regulations and protect sensitive customer information from unauthorized access and data breaches. Decentralized underwriting platforms may introduce additional security risks, such as smart contract vulnerabilities or cyberattack exposure. Insurers must implement robust security measures, such as encryption, access controls, and multi-factor authentication, to safeguard data privacy and integrity.
Regulatory Compliance
Compliance with regulatory requirements poses a significant challenge for insurers leveraging data and analytics in underwriting. Regulations governing data privacy, consumer protection, and anti-discrimination laws vary across jurisdictions and may be subject to frequent updates and changes. Insurers must stay abreast of regulatory developments and ensure their underwriting practices adhere to applicable laws and regulations.
Bias and Fairness
Data-driven underwriting processes are susceptible to bias, which can lead to unfair treatment of certain individuals or groups. Biases may arise from historical data patterns, algorithmic biases, or inherent limitations in data collection methods. Insurers must implement measures to mitigate bias and ensure fairness in underwriting decisions, such as regularly auditing algorithms, diversifying data sources, and incorporating fairness metrics into model evaluation.
Complexity of Analytics Models
Developing and deploying advanced analytics models for underwriting can be complex and resource-intensive. Insurers may need more in-house expertise or infrastructure to build and maintain sophisticated analytics models. Additionally, explaining the rationale behind model decisions to stakeholders, regulators, and customers may pose challenges, particularly for complex machine learning algorithms.
Data Governance and Ethics
Insurers must establish robust data governance frameworks to govern data collection, storage, and use for underwriting purposes. Ethical considerations, such as transparency, accountability, and consent, play a crucial role in data governance and require insurers to adopt ethical data practices throughout the underwriting process. Insurers must also ensure data usage complies with ethical guidelines and industry best practices to maintain trust and credibility.
Operational Integration
Integrating data and analytics into existing underwriting processes and systems may require significant organizational change and operational integration. Insurers must invest in training and upskilling employees to effectively leverage data and analytics tools and integrate them seamlessly into daily operations. Resistance to change and cultural barriers may impede successful integration efforts and require strong leadership and change management strategies.
Data Silos and Fragmentation
Insurers may encounter challenges related to data silos and fragmentation, where data is dispersed across disparate systems or business units. Data silos hinder the ability to derive holistic insights and may result in inefficiencies and redundancies in data management and analysis. Insurers must break down silos and promote data sharing and collaboration across the organization to unlock the full potential of data and analytics in underwriting.
Conclusion
Leveraging data and analytics in decentralized insurance underwriting has become essential for insurers seeking to enhance operational efficiency, mitigate risks, and deliver personalized customer experiences. By harnessing the power of data-driven insights and blockchain technology, insurers can change how insurance is underwritten, ultimately leading to a more resilient and customer-centric industry ecosystem.